Reinvent, remarket and retain your business with predictive analytics
Are you leveraging on the right marketing channels? Can you optimize your expenditure and still increase your customer retention?
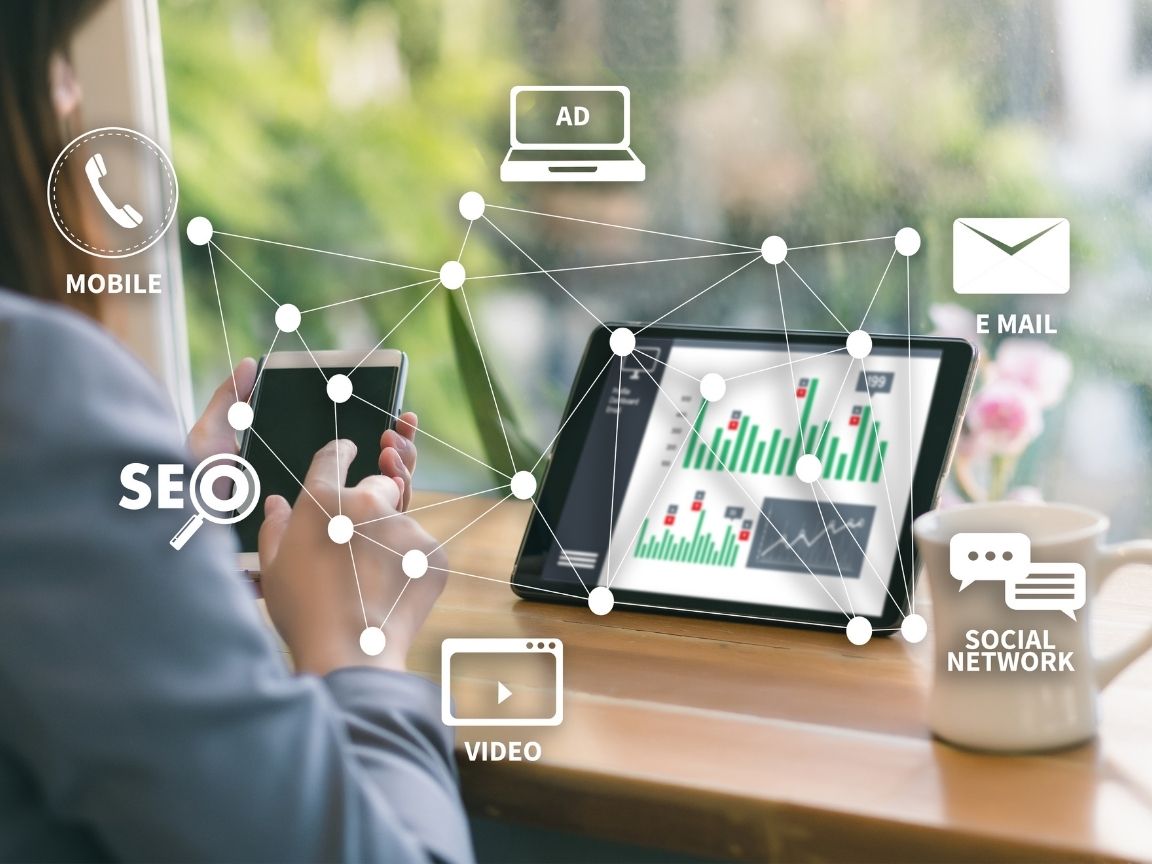
Overview
As a retail business, it is imperative to know the touch point of converting customers and analyze the best channel to achieve the same. Technology companies spend their marketing budget over a variety of marketing channels. Our client had a cosmetic business with both online and offline retail channels. Our client wanted us to model the performance of the marketing channels to derive insights about the returns from marketing investments. They wanted to model a system to analyse what set of customer actions across different channels contributed to a conversion. They wanted to optimize their marketing expenditure and efforts to achieve more sales, and therefore more profit.
Challenges
The customer's journey through multiple channels is a challenge in the perspective of data collection, particularly if the channel is offline retail. In the cosmetic industry, tracking marketing channels that influences the point-of-purchase is extremely difficult.To study the impact of each marketing channel we measured metrics like social media engagement, the click-through rate on digital advertisements, quality of queries raised on product forums, etc. We have to pull data from each channel to analyze a customer's conversion journey, and give value or credit to each. The multi-touch attribution model needs to adapt to the changing scenarios and predict the customer's journey through a conversion.
Solution
We started the data acquisition phase of the project by collecting the marketing expense records from their digital records. We also performed web scraping on the social media handle of their products to measure user engagement, product reviews, and general sentiment. We then converted this multi-feature dataset into appropriate feature vectors to make it amenable to ML training. We modeled the Machine Learning activity as a Ranking problem where the algorithm will assign ranks for the marketing channels based on the multi-feature data points pertaining to this channel. We also built a neural network architecture based on Multi-Layer Perceptron followed by a Decision Tree. It was observed that the MLP method performed the best in terms of both accuracy and execution time. We packaged the MLP based ranking model as a self-contained analytic tool that our clients can use on a periodic basis to identify the performance of the marketing channel. The analytic tool ingested the marketing expense digital records and produced reports that monitor the performance of the marketing channel.
Impact
The attribution model designed brought about a decrease in the marketing expenses by 20% and yet retain the same level of lead conversions as before. The MLP based ranking model was more than 95% accurate in its predictions. The analysis contributed to an increase in customer engagement and boosted their sales by 20%. Our predictive analytics was able to provide optimized solutions to adapt to changing customer needs. The model brought in a signigicant change in the marketing methods for channels that were not performing well.
Technology stack
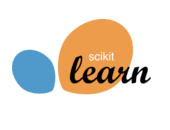
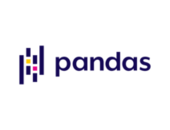
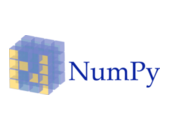
Trusted Worldwide By Innovation Driven Companies
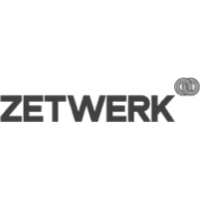
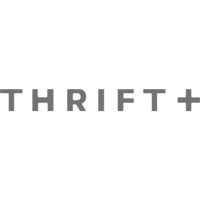
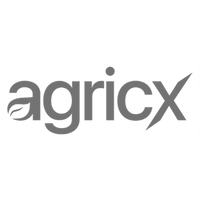
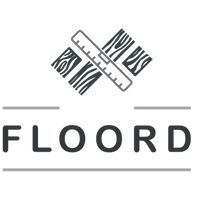
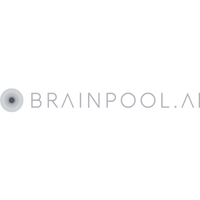
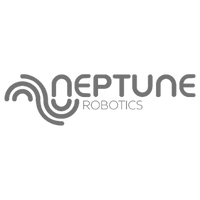
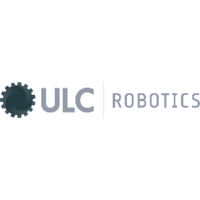
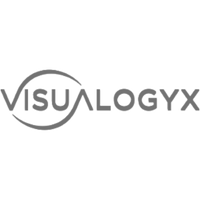