Propel your retail brand to the forefront with AI-powered customer sentiment analytics
Resonate the voice of your retail brand with that of the customer. Bring in more conversion by turning their feedback into useful insights.
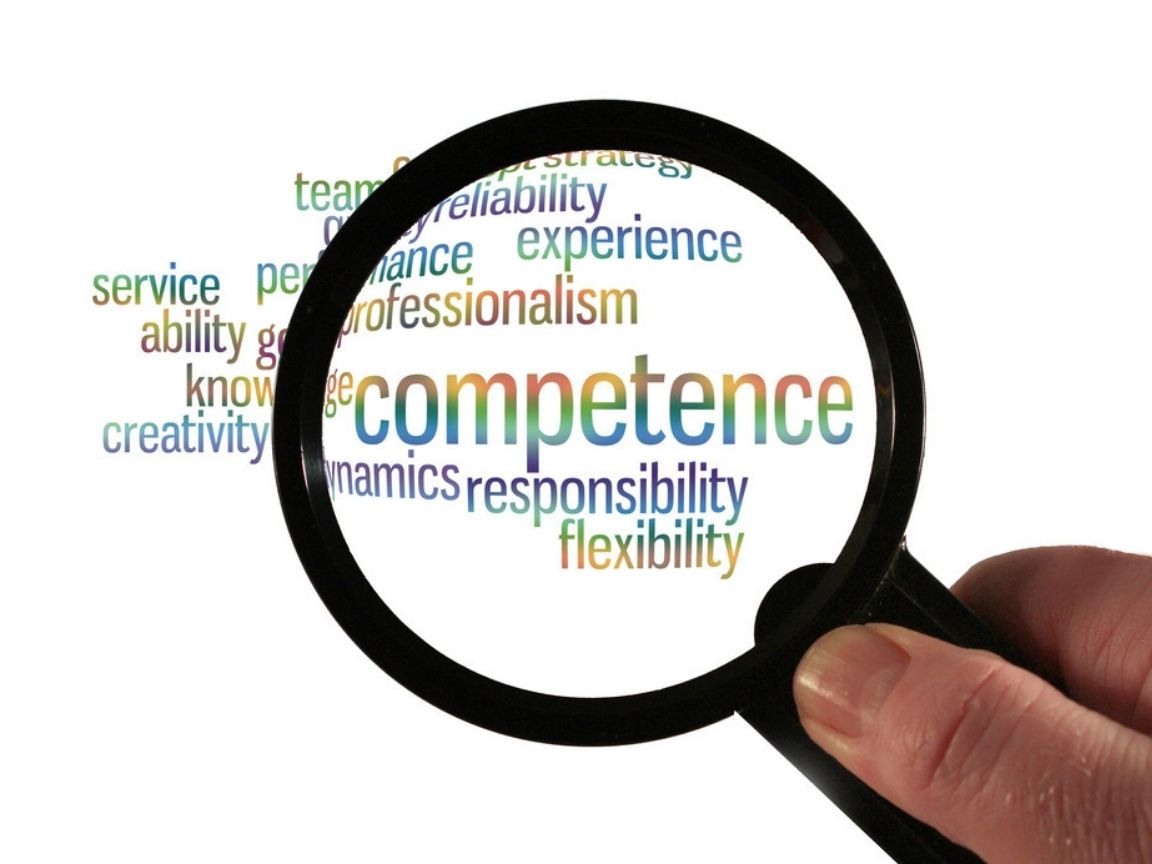
Overview
Retail businesses are required to constantly improve their products and services in order to establish their presence. Our client was a brand agency which was responsible for establishing and propelling a retail brand to the forefont in the market. Designing marketing strategies based on customer sentiment was the key approach to increase brand reach. They needed to capture the customer attention and address their concerns in a quick and efficient manner. Analyzing customer feedback and reviews manually was a time consuming and labour intensive process. It can also cause a delay in addressing urgent concerns and issues thereby causing customer dissatisfaction with the brand. Providing timely support and attention to customers are integral to building customer loyalty and retention.
Challenges
The customer feedback collection was large and to manually read or address them proved to be a humongous task. There was a compelling need to automate this process and extract useful information for feedback comments. The requirement was to quickly and efficiently to assess the feedback sentiments and address problems that were necessary. This required study and classification of the comments to categorize them and segregate them to extract useful information without spending too much time or labour.
Solution
We first started to identify positive, negative, and neutral sentiments from the feedback comments. Since the business had already accumulated a large collection of feedback comments, we proceeded directly to data curation process. In the data curation process we put the feedback comments into the three sentiment buckets using a manual process. We also used a public datasets for augmenting the clients dataset. We used unsupervised learning to convert the feedback comments into suitable feature vectors. From the feature vectors we built a multiclass classification model and the model was able to classify the sentiments with more than 90% accuracy. We then developed a reporting module to trigger alerts on the client's inbox when they received negative feedback about their service.
Impact
The strategy that we designed helped the client to derive useful insights into product performance. This proactive method of grievance redressals increased their brand reach by 15%. The client devised marketing strategies and promotion plans around the insights that we uncovered. The useful information extracted from the feedback propelled their sales by 20%. Our clients were able to trigger a significant increase in customer engagement for the brand within 3 months of engagement. Quanzig reports that sentiment analysis engagement helped a sports brand to pptimize marketing campaigns and boost sales revenue By 27%. (https://www.quantzig.com/content/sentiment-analysis-engagement-helped-sports-brand-optimize-marketing-campaigns?utm_sourceCSWeek37&utm_medium=OffsiteWeek37&utm_campaign=OffsiteCSWeek37)
Technology stack
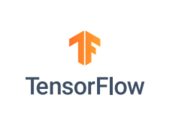

Trusted Worldwide By Innovation Driven Companies
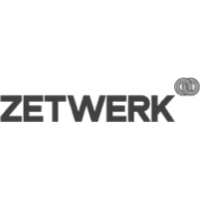
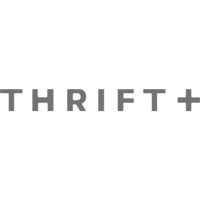
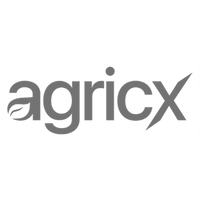
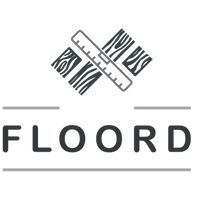
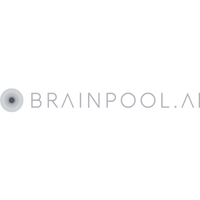
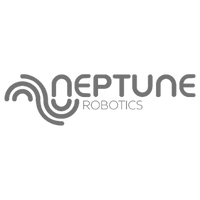
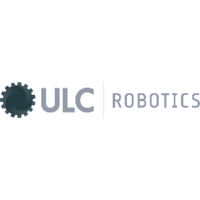
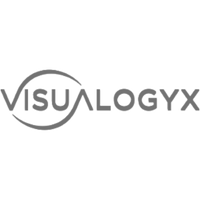