Top 5 AutoML myths that every business should be aware about
All major ML stack providers have some form of AutoML in their offering. If ML can be automated, do we really need AI engineers and data science teams ?
Gayathri Venkataraman
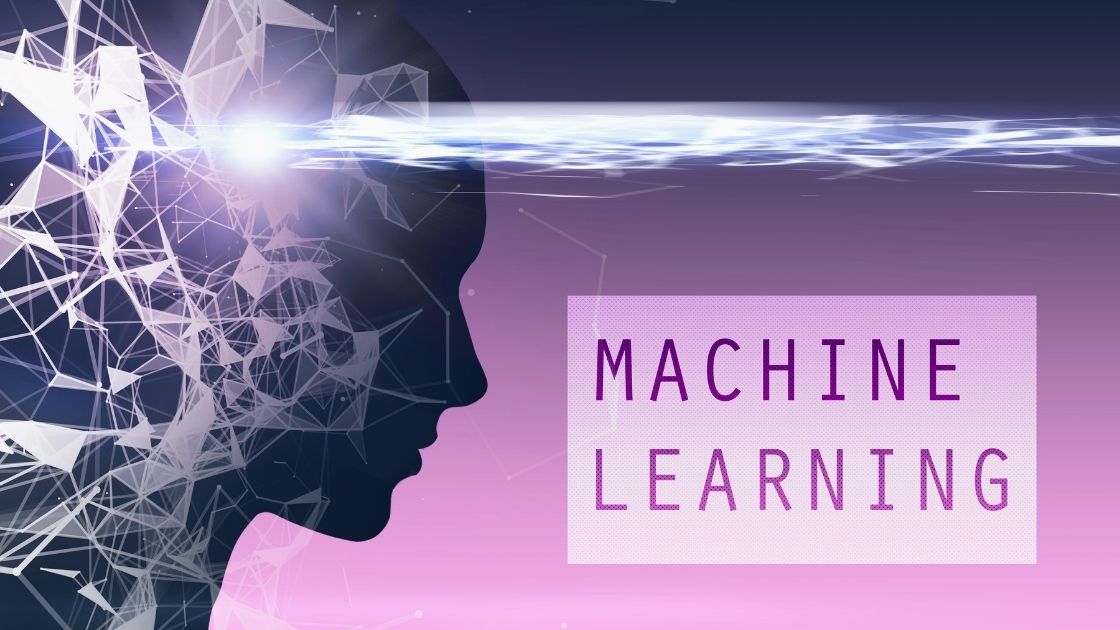
The Wikipedia definition of AutoML is Automated machine learning (AutoML). It is the process of automating the application of machine learning to real-world problems. AutoML covers the complete process from the raw dataset to the deployable machine learning model. In short, it is a technology that empowers the AI technology with AI, that is using AI to achieve AI. AutoML automates Machine Learning with a click of a button, thereby simplifying the process of algorithm implementation, research, preparation, code and pipelines. This will come as a boon to people who are new to ML and want to extract its benefits. For some, this could be a huge hindrance as their bespoke modifications will take a hit. AI by itself is a process that creates an advantage of automating processes and services in the business thereby making it smarter and efficient. So what fun do we get out of automating the AI and why do we need it?
As we have all seen and experienced over the few months, the world has changed, the way businesses are done has changed, the way people look at businesses have changed and change seems to the only thing that is permanent and unaffected. With changing scenarios, factors and changing data, the way the AI processes and ML models must also change. The current AI models may not be valid for the present pandemic situation. For example, if you look at the travel industry, the current AI models will be completely outdated as they rely on data generated from the pre-pandemic era. This data and information are no longer valid in the current pandemic scenario and the results coming out of such outdated AI models will be inaccurate. The AI that was used to make this business smart will actually bury it and make it disappear! Therefore remodelling this process, making it more responsive and suited to the current situation becomes an essential part of the business process.
New data must be collected, and the models must be trained and remodelled using this new data so that the results or predictions it produces coincides with the current scenario. Responsible AI technology always reflects the changing real-world scenarios and makes credible predictions based on it. A machine learning project involves multiple processes and stages like new data input, the data preparation process, training of the model, analysing the results, explaining and interpreting the model. Depending on the measure of the accuracy of the results, changes are made to all these stages to achieve the desired accurate results. We can see that some back and forth tweaking, changing and adjustment of values are needed to do this feedback loop. There may also be a requirement to experiment with different algorithms and different parameters to achieve accuracy. Retraining is not just feeding new data and retraining the model, it has permutations and combinations of values and parameter changes. AutoML can simplify this laborious process. Giving an AutoML process the range of values, different algorithm options and the feedback loop threshold values can make it smart. AutoML can churn out the most optimal and accurate ML model suited for the current situation.
We have seen that we can give AI powers to AI and we know what it can do. One question that pops up in our mind is that, so if all this can be automated, why do we need data scientist, engineers and why do we even need an AI team. Well, let us discuss what AI or AutoML doesn’t do. What are some of the cautions when using AutoML.
AutoML does not replace data scientists
Claims that data scientists can be replaced by AutoML is not at all true. We still need the data scientists and data science to power AI projects and take it in the right direction. All we can do is to give the data engineers more power and time to concentrate on driving these AI projects to phenomenal success.
AutoML cannot do end to end data preparation
Data is huge and massive and requires a lot of attention when building an AI product. Automating the full data preparation process completely from start to end is a huge task and is definitely not possible. There are places which require manual effort to work on the data. However, there are some parts or processes that can be automated and made more efficient.
AutoML does not replace domain knowledge
The domain knowledge is what gives a business its uniqueness and a competitive edge. Looking for projects and solutions which will direct your business knowledge and projects will make it really strong and more effective. AutoML in your domain, answering a specific problem statement is what will give you the edge over others and AutoML cannot drive your business goals or objectives.
AutoML does not by itself create an AI culture or data literacy
Finally, AutoML cannot drive an AI culture within your business. This is something you will have to put your effort on. Automating some machine learning processes in your organization will give you the time and drive to promote the AI culture in your company. You can make your organization aware of the data and processes and ensure their literacy and expertise on what is happening on the AI front in the company.
What we can learn from the capabilities of AutoML is, yes we can automate and make your smart processes even smarter but it comes with its own perks and caution. One advice experts give through their experience of AI technology is that identify your problem statement, drive your domain knowledge towards finding out how AI or AutoML will benefit your business and its customers. We just cannot use it because it can be used, it should serve a purpose and together with AI, your business should also move forward.
References
AI and AutoML: Debunking MythsTrusted Worldwide By Innovation Driven Companies
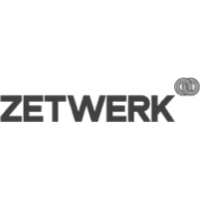
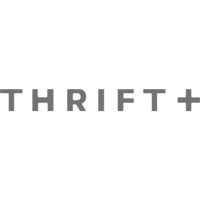
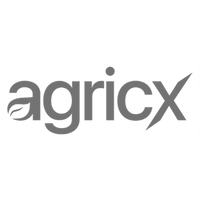
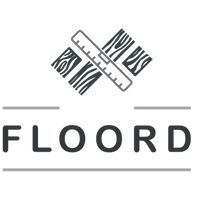
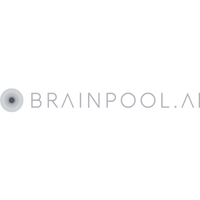
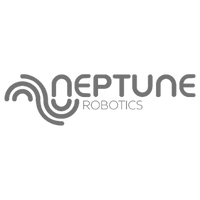
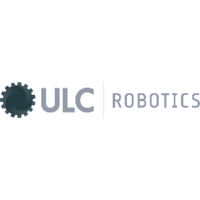
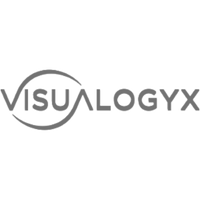